Luminance plays a central role in the astrophotography workflow. By isolating the luminance data (black and white), it simplifies processing to reveal the subtle brightness and contrast variations within deep sky objects. Luminance channels offer the richest detail in terms of brightness, making them ideal for capturing faint structures. This isolation allows the luminance workflow to tease out distant galaxies, nebulae, and other delicate features that might otherwise be lost in the color data.
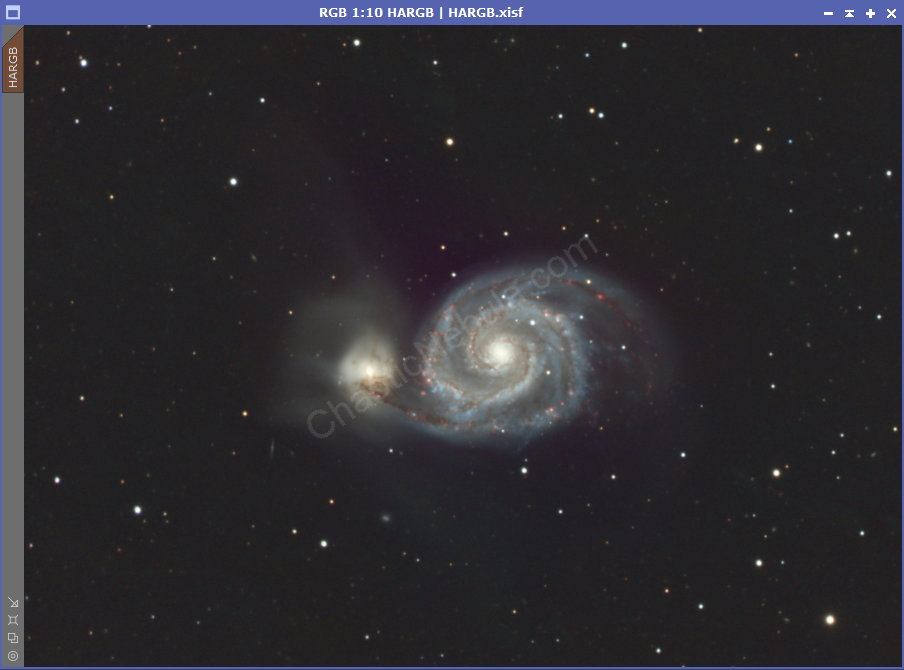
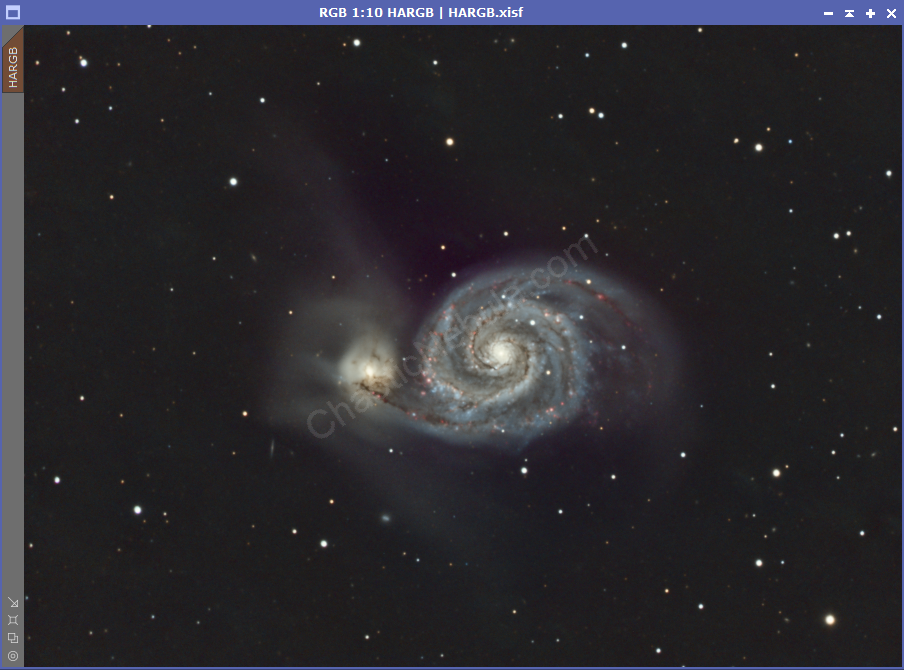
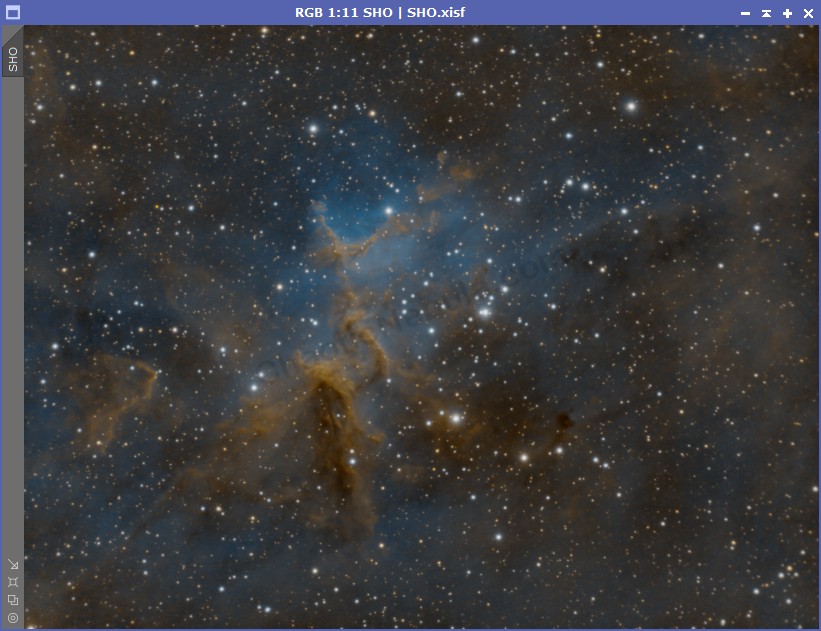
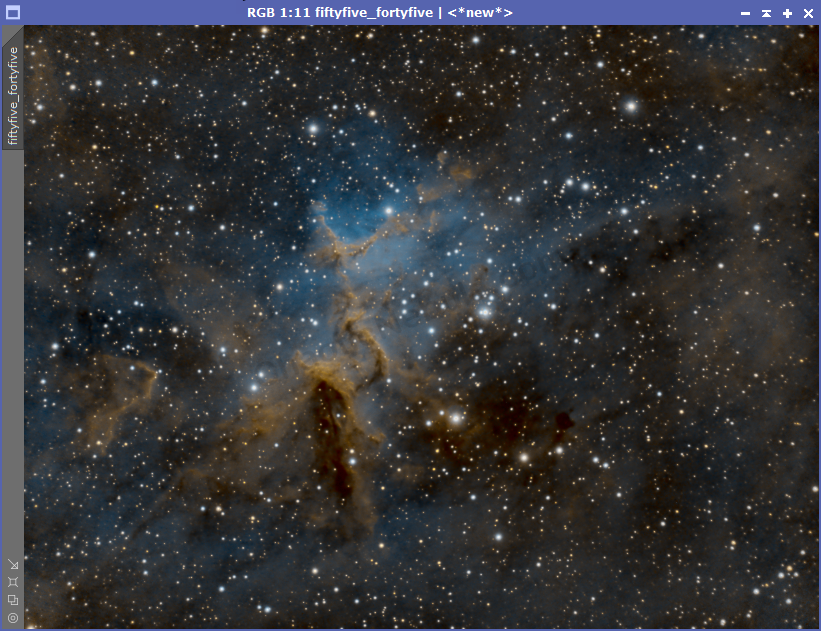
There are two ways to create a luminance channel:
- Synthetic Luminance: Extracting the luminance from a combine RGB or SHO image
- Real Luminance: Capturing a dedicated black and white image directly through the imaging rig
This workflow demonstrates how to process luminance to improve color astrophotography images.
Synthetic Luminance: Linear Processing
A synthetic luminance channel is created by extracting the L channel from an integrated image of red, green, and blue channels (Broadband) or Sulfur-II, Hydrogen-Alpha, and Oxygen-III channels (narrowband). However, a synthetic luminance might introduce artifacts or miss some of the finer details compared to a real black and white image captured directly by the camera. The benefit of using a synthetic luminance is that it saves valuable telescope time.
For creating the best synthetic luminance, use the extracted luminance channel directly after integrating your broadband or narrowband data, as detailed in the following workflows:
With the extracted luminance channel, apply the following processes.
- Star Removal: The enhancements applied to bring out subtle detail in the luminance can negatively impact the stars. By removing stars, it is easier to focus attention on the signal. For star removal, the best results are obtained with StarNet.
- Deconvolution: Deconvolution improves the clarity in high-signal areas of the image by reversing the effects of blurring introduced during the image acquisition process. (Note: If BlurXTerminator was used, skip this step as deconvolution was already completed)
Real Luminance:
Linear Processing
Unlike a synthetic luminance, which combines color data after capture, a real luminance channel is captured directly by the camera sensor as a black and white image. This dedicated approach provides the most detail for brightness information, capturing all the subtle variations in light and shadow of the celestial object. While synthetic luminance can be a time-saving alternative, the real deal offers unmatched detail and avoids potential artifacts.
The linear processing steps for the luminance channel are very similar to the linear processing steps for the color channels.
- Dynamic Crop: Removes the edges from the integrated image, as they often have missing data or very low signal.
- Gradient Removal: Helps remove background noise often resulting from light pollution or vignetting from the imaging rig.
- Noise Reduction and Deconvolution: Option 1 – PixInsight Native Processes
- TGV Denoise: Background noise corresponds to noise at the individual pixels. TGV Denoise helps remove this from your images.
- Multiscale Linear Transform: Medium-level noise corresponds to multiple pixel level noise. Multiscale Linear Transform helps remove different levels of noise at different layers
- Star Removal: The enhancements applied to bring out subtle detail in the luminance can negatively impact the stars. By removing stars, it is easier to focus attention on the signal. For star removal, the best results are obtained with StarNet.
- Deconvolution: Deconvolution improves the clarity in high-signal areas of the image by reversing the effects of blurring introduced during the image acquisition process.
- Noise Reduction and Deconvolution: Option 2 – PixInsight Paid Add-Ons
- BlurXTerminator: Leverages AI trained on high quality astronomical photos. Applies dynamic levels of deconvolution to different aspects of the image, often resulting in more natural looking and more detailed final images
- NoiseXTerminator: Leverages AI trained on a massive dataset of astronomical photos. Applies selective noise reduction while trying to minimize the impact on fine details and sharpness within the image
- Star Removal: The enhancements applied to bring out subtle detail in the luminance can negatively impact the stars. By removing stars, it is easier to focus attention on the signal. For star removal, the best results are obtained with StarNet.
Non-Linear Image Processing:
Luminance Channel
Most of the work on the luminance image happens in the non-linear (stretched) stage. This is where we can enhance details, improve contrast, and brighten faint regions.
- Luminance Stretching: Provides the first pass stretch focusing on the entire image.
- Luminance: Selective Stretch: Provides a second pass stretch focusing only on the faint areas. Useful for brightening faint structure without overexposing the dark background or brighter regions.
- Luminance: HDR Transformation: Helps recover details within the bright regions of celestial objects that might get lost after stretching the image.
- Luminance: Sharpening: The fine details within nebulae and galaxies are often obscured due to the lack of sharpness in the image. PixInsight includes multiples processes that can improve the sharpness in an image.
- Luminance: Local Histogram Equalization: Apply a targeted approach for contrast adjustment to better reveal the faint details hidden within darker regions of an astrophoto.
- Luminance: Dark Structure Enhance: Adds clarity to dark dust lanes
- Luminance: ACDNR Noise Reduction: Reduces noise in the low signal areas before integrating with the color image.
- Luminance: Integrate with RGB (LRGB): Adds the luminance channel into the previously integrated color image to help highlight the details that often get lost in the color data